Interaction prediction using GNNs
Interaction understanding and multimodal motion prediction in the self-driving domain using GNNs.
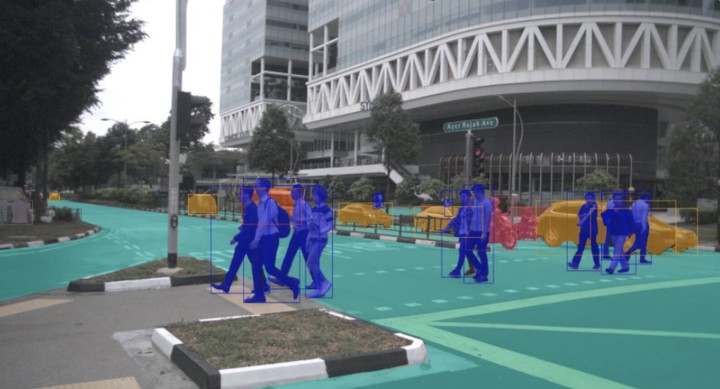
Multimodal motion prediction
A key in automotive applications is the ability to predict the future. This ability is necessary step for planning as it enables an autonomous vehicle to gauge the value of an action based on how the future evolves as the action is executed. This project focuses on predicting trajectories for other road users. One challenge is that the future is inherently stochastic. More specifically, these trajectories often exhibits multiple modes. As an example, consider a vehicle entering an intersection. The vehicle has multiple options and until the vehicle uses indicators or begins to turn, it cannot be known where it will exit the intersection.
What and why?
The aim of this project is to investigate how this uncertainty is best modelled in the context of a deep neural network making predictions about the future. The choice of modelling may have major effects on the stability during training and final performance. Furthermore, as a second direction, this research project aims to improve the explainability of the deep neural network. Predictions made in these scenarios are often highly explainable. For instance, consider the scenario of a pedestrian about to cross the road. Other vehicles tend to slow down and give way to the pedestrian. A neural network trained to predict future trajectories would likely capture this behaviour, however, it remains challenging to actually extract the explanation from the neural network. Such explanations are the secondary aim of this project.
Contribiution
Main tasks:
- model the motion prediction (multimodal distributions),
- use self supervised learning to extract more informative representations,
- explain results with GNNs.
Technologies used: Python, Pytorch
Methods used: Deep Neural Networks, Graph Neural Networks, Motion Prediction, Multimodal learning